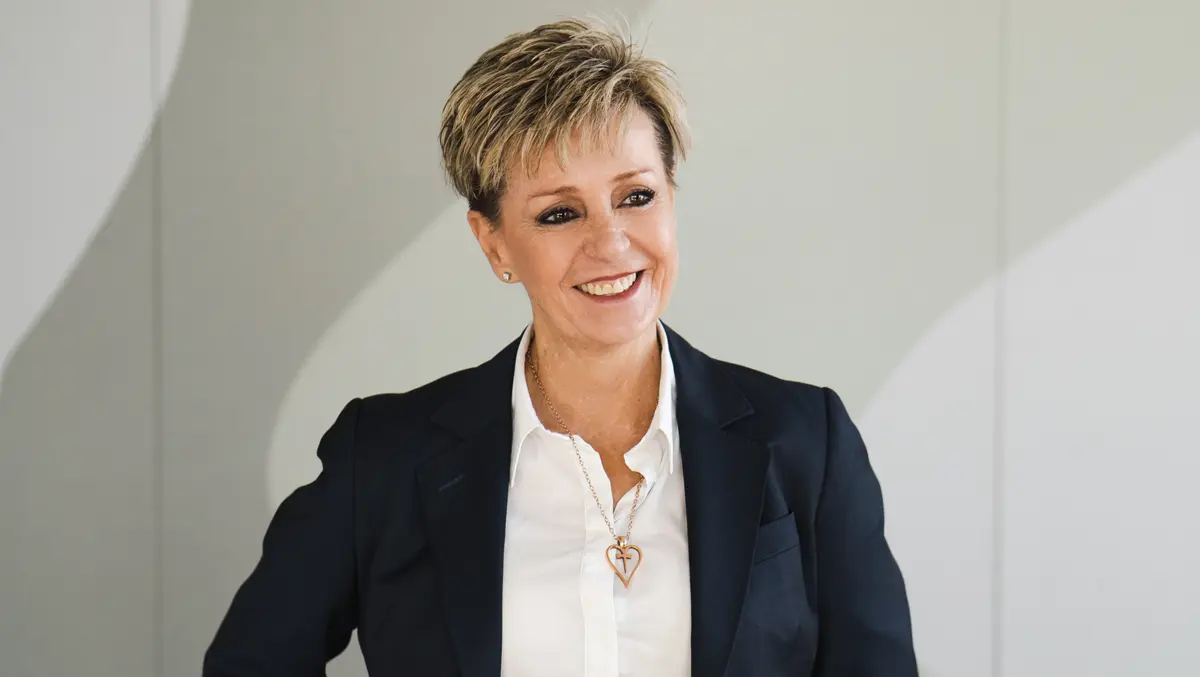
How to navigate the micro-macro dichotomy of GenAI
When generative AI entered the mainstream in early 2023, it promised to revolutionise the business landscape by transforming every facet from strategy to innovation. Yet, business leaders are still trying to understand the value and risk it introduces.
It's created a dichotomy where the macro benefits—things like productivity, resource optimisation, and cost savings—are hindered by short-term challenges in decision-making that are not only holding businesses back but also overloading them with seemingly infinite choices of where, when, and how to deploy generative AI.
Because of this, it's easy to become paralysed by the countless opportunities and challenges that generative AI presents. From challenges like ethics and regulatory implications to transformational benefits like the ability to modernise legacy code bases, there's a lot to digest, make sense of, and convert to value for businesses. But one thing is certain: boiling the ocean won't shift the needle, nor will inaction.
So, where do businesses start?
Targeted, industry-specific use cases are key
Generative AI introduces use cases unique to every business and the challenges they face in their respective industries. Choosing the right problem to fix first can pay dividends later down the track. Some of Australia's major banks are already leading by example, and many businesses can learn from their initial successes.
For example, the problem of fraud and scams continues to rise in Australia. With the Commonwealth Bank of Australia handling transactions for nine million Australians, the bank is leveraging this transaction data to improve its AI model to identify unusual patterns in transactions to help prevent such threats to customers. It's a small step in integrating AI to solve a problem, but it's producing a tangible use case that the bank can now learn from and build upon.
Elsewhere, Westpac's Growth Lab measured a 46% productivity gain after testing the impact of generative AI on software development—a result that came without the loss of code quality. The study measured two groups: those who used generative AI tools versus a control group who hadn't. Moreover, 83% of junior engineers said it was aiding them to learn early in their careers, helping to lower the barrier to entry for new and aspiring tech talent at a time when the economy is crying out for more of these professionals.
Building on this, generative AI coding tools are also poised to help newer generations of software developers translate COBOL—a legacy code base known best by a generation of retiring software engineers—to newer, more agile software development standards. Not only is this helping the next generation of software engineers maintain and migrate the code base relied upon by Australia's major banks, it also allows them to do so in a way that upholds compliance.
Additionally, we're seeing industries that have typically been slower to adopt new technology–such as construction, engineering, and legal—challenge these norms and embrace AI with focused applications. For example, John Holland, a leader in Australia and New Zealand's construction industry, has equipped all employees with a private ChatGPT solution so they can write and summarise content, such as project briefs, faster.
These are just some examples illustrating that, by starting small and with a problem specific to an industry, businesses can begin to make progress with generative AI in a meaningful and impactful way. The key is to start small and build from there.
Translating micro problem solving into macro benefits
Micro-level automation and efficiency improvements contribute to macro-level productivity gains across an entire organisation. At scale, this extends to an entire industry—and further, the economy. At the same time, micro-level improvements in customer interactions and personalisation contribute to positive impacts on brand reputation, customer loyalty, and market positioning.
We're seeing this reality unfold in the world of software. As evidenced by the widespread adoption of generative AI tools like GitHub Copilot, AI has begun to rapidly refound software development, fueling up to 55% gains in developer productivity. Developers are now building high-quality products and pushing them to the market quicker than their competitors. But that's just the beginning–these productivity benefits have a ripple effect, with developer AI tools alone expected to boost global GDP by $1.5 trillion USD by 2030.
The journey of integrating generative AI begins with recognising its dual potential to transform individual tasks and entire operational landscapes. Businesses that can navigate this micro-macro dichotomy, prioritising immediate, actionable goals while keeping an eye on the broader horizon, will not only thrive but also set new standards for innovation and efficiency in their respective industries. What's crucial now is how quickly these organisations can step into the age of AI. The first to do so will exist in a different spectrum of productivity entirely, shipping ahead of the curve and delivering products to market faster than their competitors. Those who don't, will simply fall behind.